Creating Synthetic Data out of Real Data with Desired Demographics
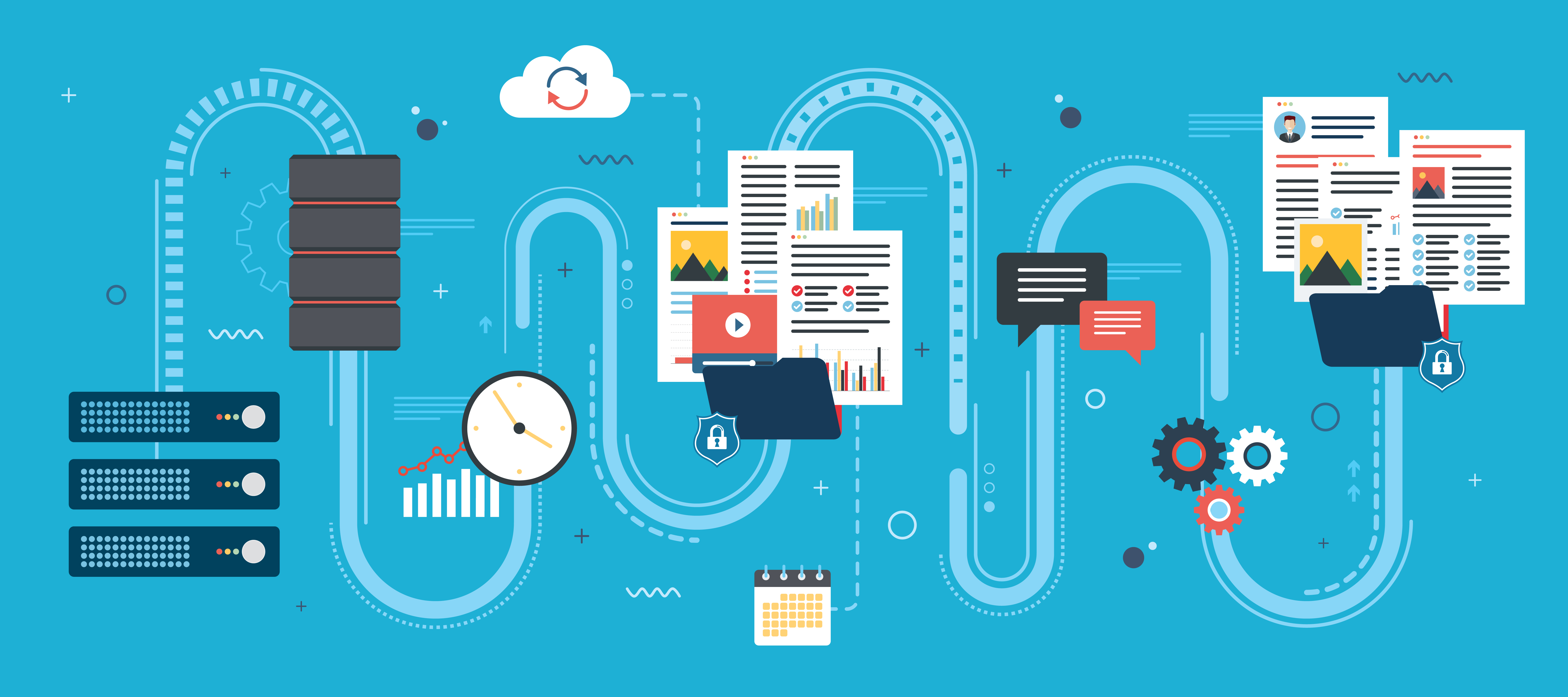
Statista Research Department in a report dated Sep 8, 2022, estimates that the total amount of data created, captured, copied, and consumed globally by 2020 was 64.2 zettabytes. Over the next five years up to 2025, global data creation they project to grow to more than 180 zettabytes.
Organizations collect this data for various purposes:
- Market Segmentation - going after new markets where you don’t have a presence
- Financial Analysis – Who are your high paying customers and what are their buying patterns
- Consumer Analysis – What are your customers buying and what are their buying patterns
- Vendor Analysis – How are your vendors performing? How is your supply chain working?
The production data you have may be inadequate for the above purposes for the following reasons:
- Inadequate Representation of Market Segments – Your products appeal to people ages 17-34 but you want to expand into other markets such as the 35-50, 51-80 age segments. You may have Gen Z customers, but you want to expand into Gen X and Baby Boomer markets. You have some data for those age segments, but not enough.
- Incomplete Data in your Databases – Your software or applications that you use may have been upgraded with new versions over time. New tables and columns may have been added. The older data may have NULL values or be empty for the newly added or changed columns.
- New Products Not Yet Established – You may have introduced new products recently for other market segments. You may have some data, but not enough as the data you have for some other segments.
- Sensitive Data – You may have the data for these other segments, but you work with external marketing and advertising agencies. You are hesitating to share it with them for competitive or intellectual property reasons.
In all the above cases, generation of Synthetic Data from your existing Real Production Data may solve the problem in the following ways:
- Using Existing Data to Extrapolate – Using Machine Learning, you can expand the data you have and augment it. Newly generated Synthetic Data can be made to have the same characteristics as those segments.
- Backfilling NULL or Empty Values – If columns contain NULL or Empty values, Synthetic Data Generation can use existing data for those columns to extrapolate and backfill those columns.
- Creating New Data from Existing Data for New Segments – For new market segments where you have data, you can use that to create new Synthetic Data that has the same characteristics.
- Masking Sensitive Data – Synthetic Data Generation can replace Personally Identifiable Information (PII) from your current data with data about non-existent people. This can render sensitive information safe to share with Marketing and Advertising consultants or others you are wary of sharing real data with.
Real Production Data that you have can prove to be inadequate for various purposes be it Marketing, Financial, Organizational, or internal or external testing. Synthetic Data generation has the potential to address concerns you have safely and effectively by augmenting your production data with new data that has the same characteristics.
Before you start your research, you need to know where to look for that data. ― Pooja Agnihotri, Market Research Like a Pro